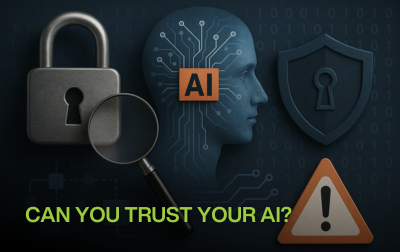
How Secure Are AI-Based Systems in Today’s Digital Landscape?
Artificial intelligence (AI) is transforming industries, streamlining processes, and opening up new possibilities across sectors. But as AI becomes increasingly embedded in business operations, questions around AI security are becoming more urgent. Can AI systems be trusted? How vulnerable are they to manipulation? And what are the implications of AI-generated threats like deepfakes and synthetic data?
This article explores the landscape of AI security, helping business decision-makers understand where the real risks lie and how to navigate them.
The Growing Role of AI in Business
AI technologies, from machine learning algorithms to natural language processing and computer vision, are being adopted to improve efficiency, automate decision-making, and drive innovation. Whether in healthcare, finance, manufacturing, or logistics, AI systems are analyzing vast datasets and making real-time recommendations. But the very qualities that make AI powerful also make it a potential target for misuse.
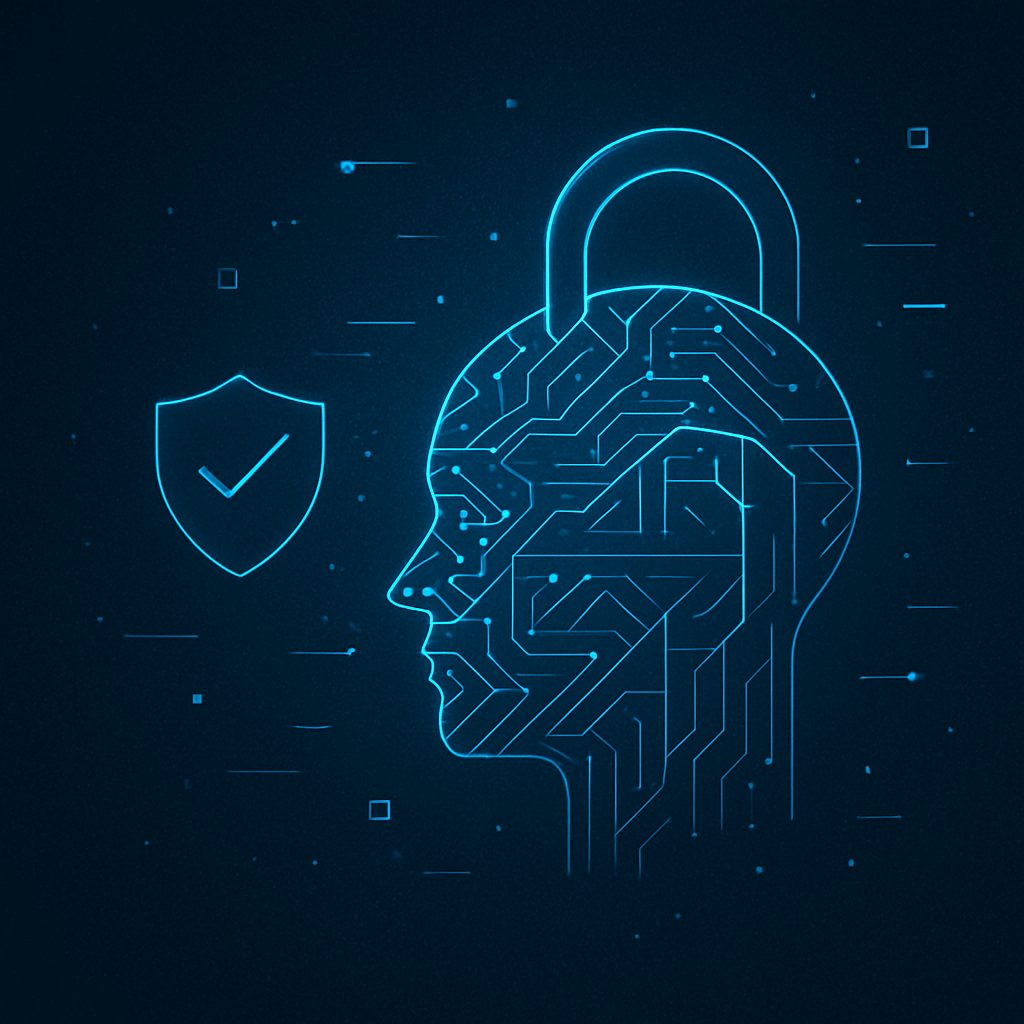
Key Vulnerabilities in AI Systems
Despite their sophistication, AI systems are not immune to exploitation. Below are several common and emerging vulnerabilities that businesses should be aware of.
Adversarial Attacks
One major concern in AI security is adversarial attacks. These involve feeding maliciously crafted data to an AI model to manipulate its behavior or outputs. For example, a seemingly innocuous change to an image could trick a computer vision system into misclassifying objects or people. In the case of facial recognition, an attacker might alter their appearance in subtle ways to evade detection or impersonate someone else. Such attacks expose the fragility of even the most advanced AI models when operating in uncontrolled environments.
Model Theft and Intellectual Property Risks
Another pressing threat is model theft. If attackers gain access to an AI model, they can clone it, reverse-engineer it, or use it to infer confidential training data. This kind of theft not only results in intellectual property loss but also poses compliance and privacy concerns. For businesses relying on proprietary AI solutions, model security is a cornerstone of their competitive advantage.
Data Bias and Unfair Outcomes
AI systems can also produce biased or skewed results if trained on unrepresentative or imbalanced data. This can lead to unfair decisions in high-stakes areas such as recruitment, lending, and healthcare. Bias in AI isn’t always malicious, but it can be just as damaging. Detecting and mitigating bias requires continuous audits, diverse datasets, and ethical review frameworks.
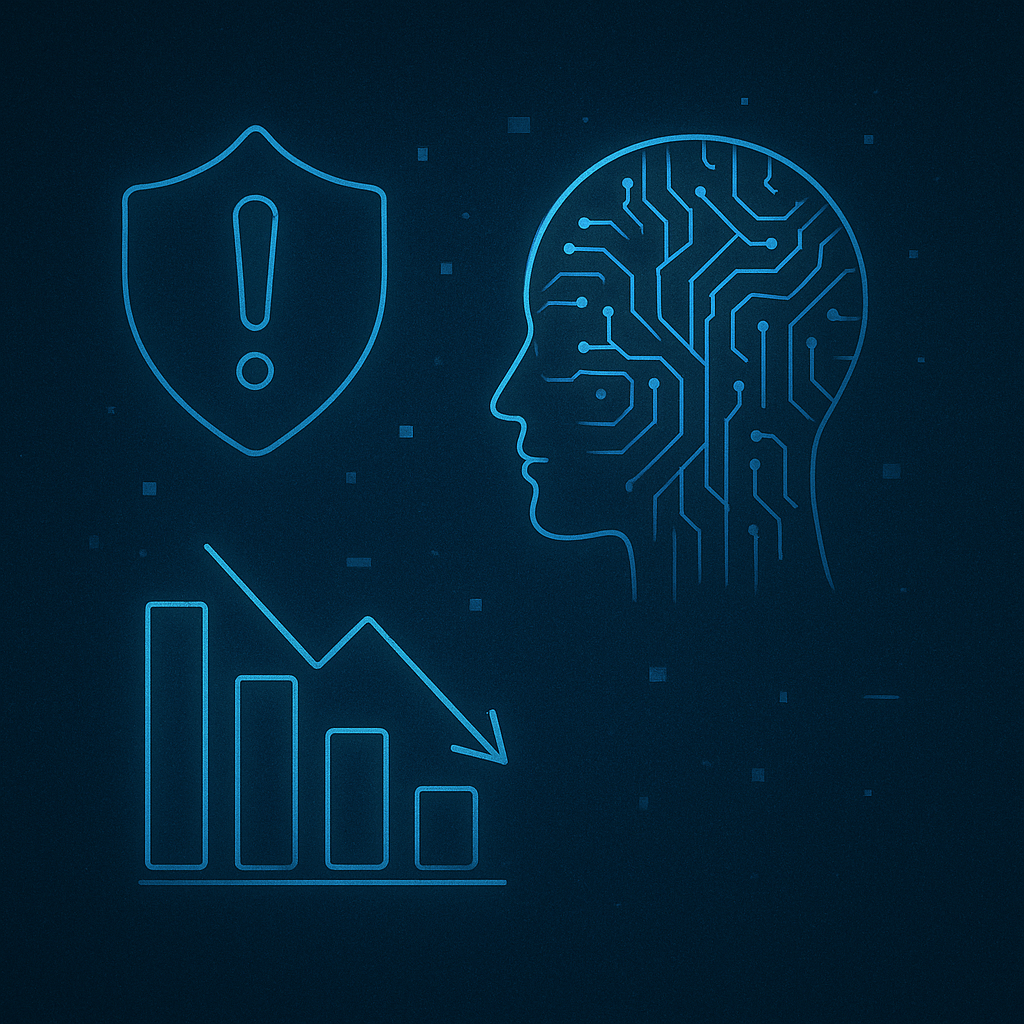
Deepfakes and AI-Driven Disinformation
Among the most visible and rapidly evolving threats are deepfakes: hyper-realistic yet completely fabricated audio and video content generated by AI. These can be used to impersonate public figures, disseminate fake news, or carry out fraud.
In the business context, deepfake technology has already been weaponized. For instance, cybercriminals have used AI-generated voices to imitate company executives and trick employees into transferring funds to fraudulent accounts. These tactics combine psychological manipulation with technical precision, making them difficult to detect with traditional security tools.
The consequences go beyond financial loss. Deepfakes can erode public trust, compromise executive credibility, and create reputational crises for companies unprepared to respond.
Synthetic Data and the Risk of Fabrication
AI can also generate synthetic data, which is artificially constructed to simulate real-world data. While useful in many contexts—such as training AI models without violating data privacy laws—synthetic data can be misused.
The Double-Edged Sword of Synthetic Data
On one hand, synthetic data helps businesses innovate faster by removing bottlenecks associated with real data collection. On the other hand, bad actors can use this technology to fabricate entire datasets that appear legitimate. These manipulated datasets can mislead AI systems, falsify performance metrics, or influence automated decisions in harmful ways.
This risk is particularly pronounced in industries that depend on data integrity, such as finance and healthcare, where decisions have immediate and serious implications.
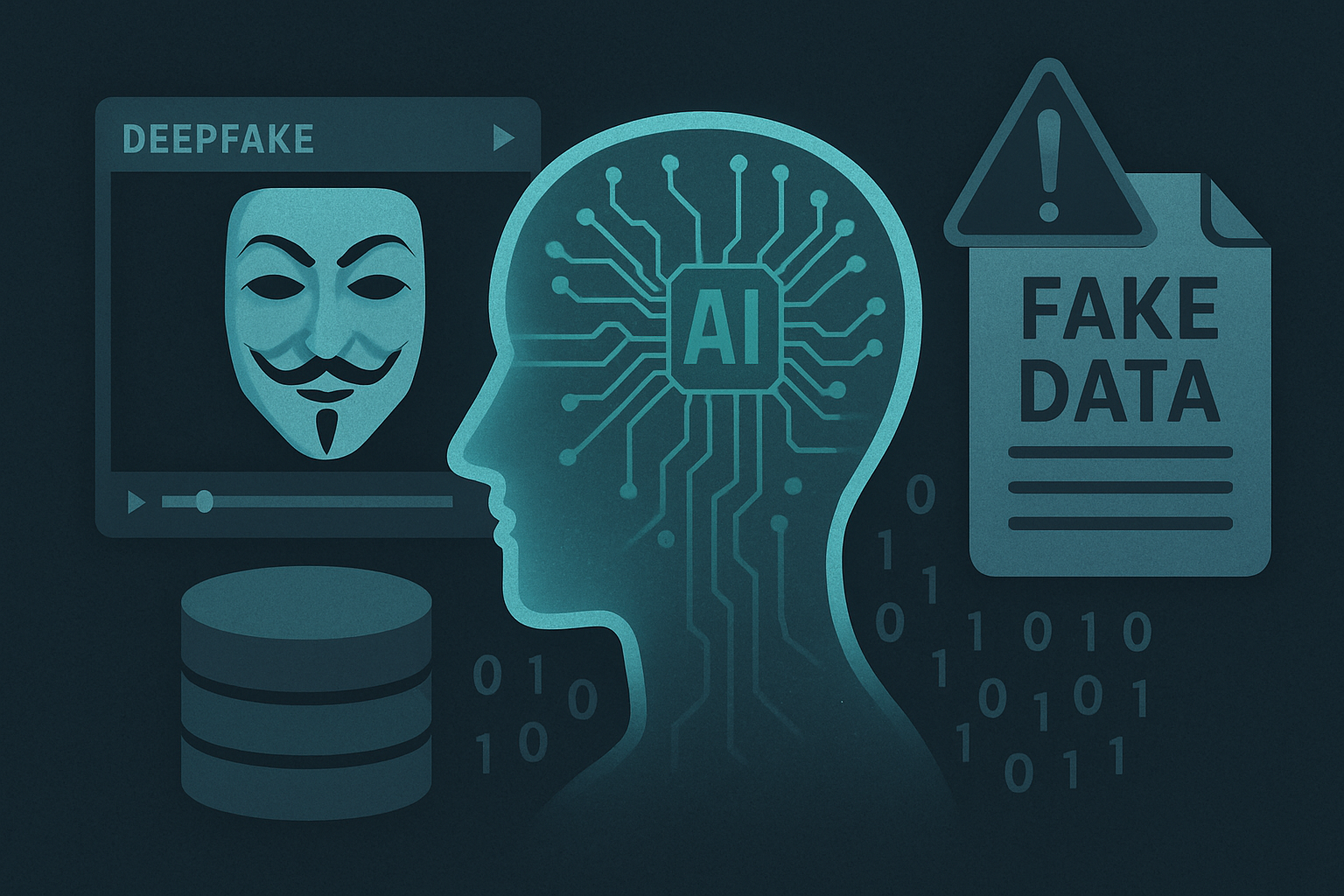
Strategies for Strengthening AI Security
Mitigating the risks outlined above requires a holistic, multi-layered approach to AI security. Businesses must embed security measures at every phase of the AI lifecycle—from data collection to model deployment.
Robust Testing and Validation
AI models should be rigorously tested against adversarial scenarios and unexpected inputs. Stress testing helps identify potential weaknesses before models are deployed in live environments. This process should include simulated attacks and edge-case analysis.
Data Integrity Controls
Maintaining high data quality is essential. Businesses should implement automated monitoring tools to detect anomalies, prevent tampering, and verify data provenance. This helps ensure that AI models are learning from trustworthy information.
Access Restrictions and Model Protection
Restricting access to AI models and training datasets is critical. This can involve encryption, API rate limiting, and authentication protocols to ensure that only authorized personnel and systems can interact with AI assets.
Continuous Monitoring and Auditing
Once deployed, AI systems should be continuously monitored for signs of malfunction or compromise. Anomalies in output or system behavior should trigger alerts and initiate predefined response plans. Regular audits also help maintain compliance with industry regulations.
Transparency, Governance, and Accountability
Finally, businesses should adopt frameworks that promote explainability and traceability in AI. Clear documentation, model cards, and version control are essential to understanding how AI decisions are made—and holding systems accountable when things go wrong.
The Role of Human Oversight
Despite advances in automation, human oversight remains essential. AI should augment, not replace, human judgment. Business leaders must stay involved in setting objectives, selecting training data, and interpreting model outputs. This is especially important in sensitive areas where ethical implications and legal exposure are high.
Investing in explainable AI (XAI) tools can bridge the gap between technical complexity and stakeholder understanding. These tools help stakeholders assess the reasoning behind AI decisions, build trust, and intervene when necessary.
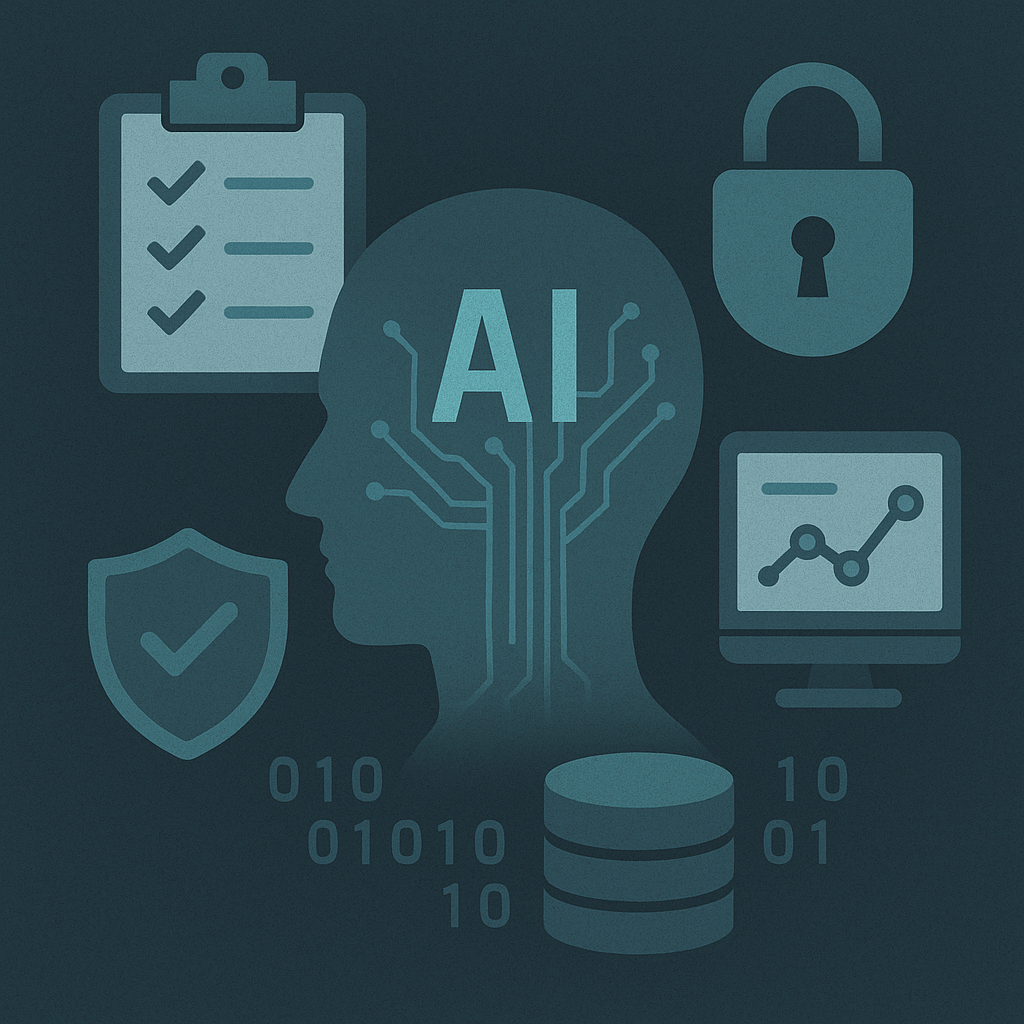
Preparing for a Resilient AI Future
AI security is not a static challenge. As AI evolves, so do the threats. Businesses must adopt a forward-looking mindset, continuously evaluating their AI assets, upgrading security protocols, and educating teams on emerging risks.
Collaboration is key. By partnering with experienced AI and cybersecurity experts, companies can stay ahead of threats and build systems that are not only powerful but also secure and responsible.
At Aleron IT, we support businesses in building secure, ethical, and high-performing AI solutions tailored to their goals. Our team ensures that every AI implementation aligns with the latest best practices in security, compliance, and transparency.
Are you considering AI integration or looking to improve the security of your existing systems? Contact Aleron IT today to ensure your AI strategy is both innovative and secure.